Subscribe to Our Newsletter
Sign up for our bi-weekly newsletter to learn about computer vision trends and insights.
alwaysAI’s One-of-a-Kind modelIQ Tool Sets the Standards for AI Model Evaluation
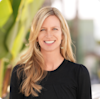
Kathleen Siddell
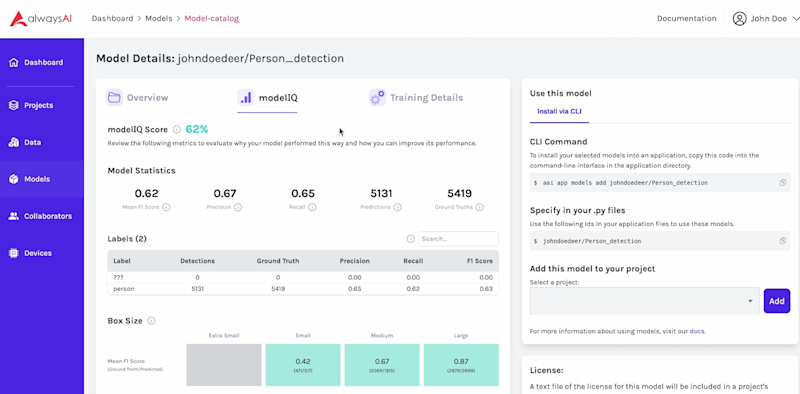
A computer vision model is the engine of the application. Just as a car can’t run without an engine, a computer vision application can’t run without a model. Proper training is essential.
Along with alwaysAI's new and improved MLOps capabilities, we are excited to offer a one-of-a-kind advanced model evaluation tool, modelIQ.
Our MLOps tools — Model Training and modelIQ — provide the fine-grained control of your model configuration and unparalleled insights into how well your model is performing. These new and improved tools give you the data you need to make decisions about your data and your training settings in order to improve model performance.
As the growth of AI continues to skyrocket, MLOps is growing more and more important. MLOps, short for Machine Learning Operations, helps to streamline the process of taking machine learning models to production, controlling versions, and analyzing their performance.
While many computer vision platforms can help you train models, there are not many that can offer true MLOps. As a truly end-to-end platform, alwaysAI can manage the entire computer vision lifecycle from collecting and annotating data through deployment, monitoring, and maintenance of your computer vision models and applications.
The addition of our improved Model Training and brand new modelIQ tool solidifies alwaysAI as a premiere MLOps provider.
What is alwaysAI’s modelIQ?
Model training can feel like a bit of a mystery. You annotate carefully, set your training parameters, and hope for the best. Unfortunately, sometimes “the best” is pretty poor – leaving you scratching your head wondering why. modelIQ takes the mystery out of model training.
modelIQ provides alwaysAI users with an overall model evaluation score broken down into several key components (like label, quadrant, and bounding box size) for an unparalleled view of how well the model performed.
Using the principles of the Confusion Matrix, alwaysAI’s modelIQ aggregates true positives, false positives, false negatives, and true negatives to determine the precision, recall, and f1 score of your model. These measures are further categorized based on the characteristics of your predictions to deliver incredibly detailed insights into how your model performs by image, label, quadrant, and size.
modelIQ is designed to help you sharpen and refine your model exactly where it's needed, saving you invaluable time and eliminating unnecessary frustration. No other tool provides this precise level of detailed information critical to optimizing model performance.
Features of alwaysAI's modelIQ
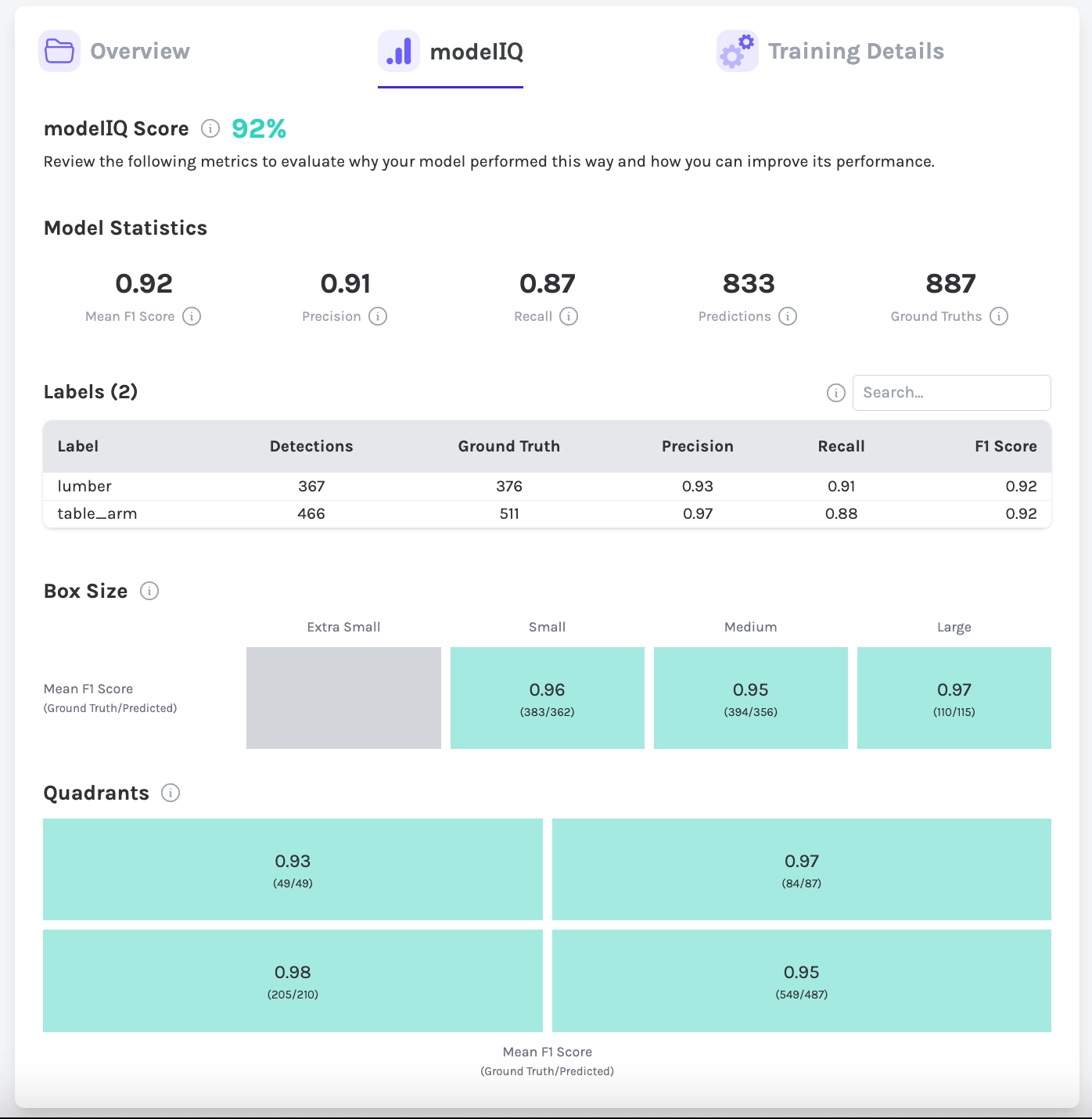
alwaysAI's modelIQ dashboard
Overall modelIQ Score
The first thing you’ll notice in modelIQ is your overall modelIQ score. This number appears as a percentage to help you evaluate the overall performance of your model. This score is determined using the following metrics: predicted/ground truth detections, precision, recall, and mean F1 score.
- Predicted Detections & Ground Truth – refers to the expected number of detections. In many cases, ground truth labels must be prepared by humans. To measure the quality of your model, we compare the predictions produced by the model with ground truth labels.
- Precision – quantifies the number of correct positive predictions out of positive predictions. Look to precision when you want to minimize false positives.
- Recall – quantifies the number of correct positive predictions out of all possible predictions. Look to recall when you want to minimize false negatives.
- Mean F1 Score – gives a generalized assessment of the model’s performance. It is calculated from the number of correct predictions (True Positives) and incorrect omissions (False Negatives) made across the entire dataset.
Breaking Down modelIQ
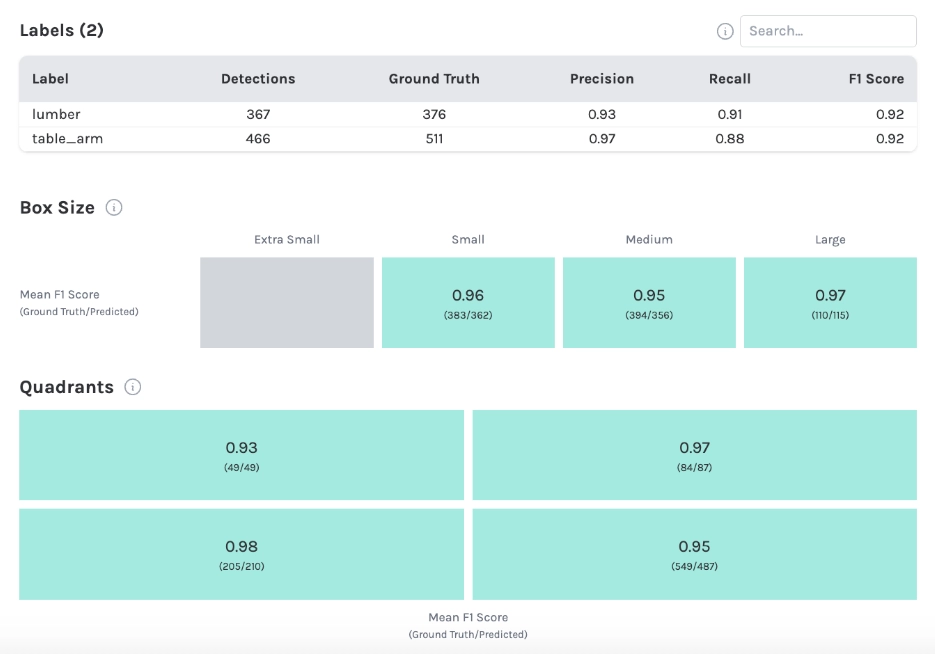
alwaysAI's modelIQ details
Not only do you get an overall modelIQ score based on these metrics, but you’ll also see a more detailed breakdown of your model’s performance by label, box size, and quadrant for your test dataset.
- Label - Some of your labels may be performing better than others. Get a detailed breakdown of this performance and leverage this knowledge to improve your model.
- Box Size – refers to the size of the bounding boxes in your model. Depending on your architecture and dataset distribution, your model may perform better or worse on differently-sized objects. modelIQ provides you with that data.
- Quadrant – refers to the area of a photograph split into fourths. Mainly for static scenes, this will help you identify whether there are weaknesses in particular areas of your environment.
Because modelIQ calculates an F1 score broken down by object characteristics, you can better understand the critical details that impact model performance. By parsing out F1 scores for each label, you can determine if any classes in your dataset need further refinement. Armed with more information, you can optimize adjustments to your dataset and your model, reducing the overall time it takes to generate your model.
Training Details
Training a production-level model is a long and iterative process. Keeping detailed records makes everything a lot smoother. The training details page is a permanent record of the configurations you used for training so you don’t have to worry about wasting cycles repeating settings. This includes standard training configurations such as epochs and batch size, as well as more advanced hyper-parameters.
Each of these hyper-parameters provides valuable information about how your model was set up, so you can understand your model on an incredibly detailed level. This is especially helpful if you are collaborating and did not originally configure the model training.
On the model details page, you’ll also see the CLI command to use to add your model to your application, an explanation of where to insert your model ID in your application file, and a dropdown menu of all your projects to conveniently add your model to an existing project.
Advantages of modelIQ
We created modelIQ to give our users critical insights into model performance.
Because models play such a critical role in computer vision applications, knowing where your model performs well and where it needs adjusting is invaluable. With modelIQ, you get the level of detail you need (in addition to these other advantages):
Greater precision in model evaluation
modelIQ takes the guesswork out of model performance. By getting specific, measurable feedback about each attribute of your model, you can assess and adjust your model with greater precision than ever before.
Faster training
Because you can parse out model performance on a more granular level, you can save time making unnecessary adjustments and focus on changes that will improve model performance with a higher degree of certainty. This will improve overall model training times so you can deploy faster.
Bring your own model to alwaysAI for performance insights (*coming soon*)
We understand that as the growth of computer vision models soars, you may have created and trained your model elsewhere. Therefore, we did not want to wait to announce we are working on “bring your own model” capabilities (BYOM). We are putting the finishing touches on this feature, so you can take advantage of modelIQ no matter where you did your model training (on or off the alwaysAI platform). Stay tuned!
Ready to Get Started?
Computer vision isn’t easy but alwaysAI aims to remove some of the complexity so all users (no matter their level of engineering expertise), can leverage the power of computer vision solutions.
Our platform is designed to take your project from start to finish with the most comprehensive tools available. From creating datasets through deployment, let alwaysAI impress you with our advanced capabilities. Give us a call today to speak to an AI expert.
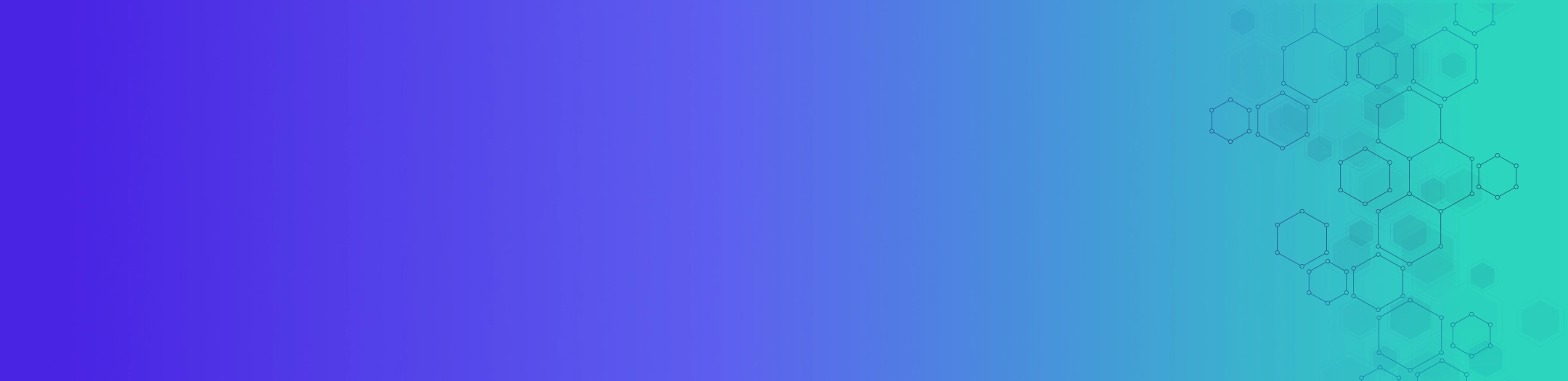